Machine learned interatomic potentials for modeling interfacial heat transport in Ge/GaAs
Abstract
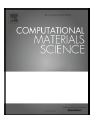
Molecular dynamics simulations provide a versatile framework to study interfacial heat transport, but their accuracy remains limited by the accuracy of available interatomic potentials. Past researchers relied on simple analytic potentials and the use of mixing rules to model interface systems, but with minimal justification for their use. Contemporary researchers have access to highly flexible machine learned interatomic potentials (MLIPs), yet these remain understudied within this problem domain. In either case, efforts are needed to rigorously assess and validate interatomic potentials prior to their use in MD simulations of interfacial heat transport. Here, we pursue these efforts while developing interatomic potentials for a model Ge/GaAs system. We first assess the quality of ab initio harmonic force constants (IFC2s) obtained from the interface structure that is used to generate fitting data for MLIPs. We show that these force constants can converge to near bulk-like values within 1 nm away from the interface while also exhibiting a complex relationship across the interface, which likely precludes any successful application of mixing rules. Subsequently, we develop two different MLIPs to model Ge/GaAs using the linear spectral neighborhood analysis potential (SNAP) functional form: one standard SNAP fit to total forces, and another hybrid SNAP fit to anharmonic force components and combined with a harmonic Taylor expansion potential. Each potential is evaluated with respect to bulk thermal properties, interface IFC2s, and stability considerations, providing a valuable comparative analysis that can guide future work.